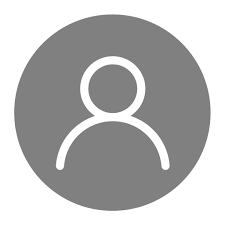
Claim denials create a significant burden on the revenue cycle. A recent report from Becker's shows that commercial claims denials jumped by 20% in just one year. For providers, recovering lost revenue from denied claims is crucial yet complicated. However, the good news is that up to 85% of denials are preventable through accurate coding, timely submissions, and thorough documentation.
This is where claims analytics plays a transformative role. By converting complex data into actionable insights, it helps streamline RCM, empowering providers to minimize errors and prevent revenue loss. This blog explores how claims analytics can optimize claims, reduce fraud, improve transparency, and guide you in choosing the right tools to help your organization succeed.
What is claims analytics?
Claims analytics involves analyzing and interpreting data from insurance claims. It gives vital insights to healthcare providers by analyzing services provided to patients, diagnoses detected, procedures suggested, and more. It also has the capability to highlight areas for improvement within the revenue cycle, enabling healthcare providers to improve operational efficiency, enhance patient satisfaction, and boost financial performance. In addition, claims analytics also aids healthcare providers in implementing targeted interventions, streamlining workflows, and enhancing claims submission processes.
What information is included in claims analytics?
Claims data includes information that provides a comprehensive view of each patient's journey through the healthcare system. Here’s what claims analytics typically includes:
Diagnosis codes
These codes indicate a patient's medical condition and form the basis for determining necessary treatments and associated costs.
Procedure codes
Procedure codes outline the services and treatments rendered, allowing healthcare providers and payers to assess resource utilization and billing accuracy.
Patient demographics
It consists of age, gender, location, and socioeconomic data that help healthcare providers create personalized treatment options and identify at-risk populations.
Provider information
Data on healthcare providers, including specialties and locations, helps track variations in care and identify patterns in service delivery.
The technology behind claims analytics
To unlock the full potential of claims analytics, healthcare providers must understand how technologies such as artificial intelligence (AI), machine learning (ML), and data mining contribute. These technologies extract and interpret complex datasets for seamless claims analytics.
Artificial intelligence
AI algorithms automate and streamline the claim analysis process, reducing manual effort and accelerating actionable insights. AI also assists in identifying billing errors and anomalies in real time, ensuring discrepancies are flagged before they lead to denials.
Machine learning
ML models learn by analyzing historical claim data to identify patterns and make accurate predictions. By learning from past denials, ML can help prevent claim denials by forecasting which claims are at risk of rejection. This helps providers proactively address issues and optimize approval rates.
Data mining
Data mining techniques deep-dive through claims data to discover hidden patterns. Data mining in claims analytics allows providers and payers to identify factors contributing to recurring denials and revenue leakages. It identifies the root causes of denials, empowering organizations to address systemic challenges and prevent revenue leakages.
Benefits of claims analytics
There are numerous benefits of claims analytics. Healthcare providers can achieve financial excellence, increase operational efficiency, enhance patient care, and stay compliant. Here’s how:
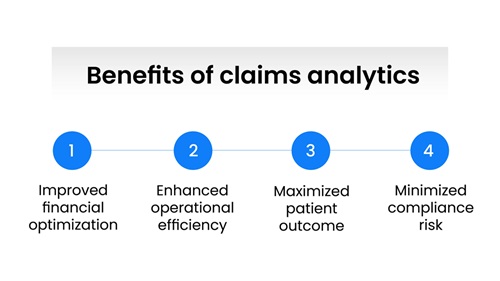
Improved financial optimization
Claims analytics identifies common reasons for denials, medical coding errors, billing issues, and more, helping healthcare providers spot patterns in reimbursed and denied claims. Claims analytics can also help automate significant parts of the billing process, efficiently improving cash flow and lowering administrative costs.
Enhanced operational efficiency
Providers can improve their operational workflows by analyzing claims data. They can pinpoint areas in the revenue cycle where delays or errors are common. Data-driven insights also refine processes to reduce claim processing times, from submission to payment. Faster turnaround times mean that providers can allocate resources more effectively.
Maximized patient outcomes
Claims analytics helps identify trends in patient health conditions and common comorbidities. This information can be used to develop preventive care programs, reducing the risk of high-cost treatments and hospital readmissions. Providers can understand which treatments are most effective for specific patient demographics, conditions, or health histories and offer personalized treatment to them, increasing patient outcomes and satisfaction.
Minimized compliance risk
Claims analytics tools can flag non-compliant claims and identify gaps in RCM practices. This helps healthcare providers enhance regulatory compliance and minimize financial risks. Practices could know potentially fraudulent billing practices or errors in the revenue cycle that may otherwise go unnoticed. Early detection of these anomalies protects providers from penalties and financial losses.
Use cases of claims analytics
Claims analytics has broad applications that help providers make data-driven decisions. Here are some key use cases:
CPT code analysis
Claims analytics helps healthcare providers identify which procedural codes encounter the most issues in reimbursement. With CPT code analysis, RCM teams can highlight CPT codes that:
- Face frequent payer denials.
- Are consistently misused, either by being unbundled improperly or applied inaccurately.
- Show unusual utilization patterns compared to industry benchmarks.
Healthcare providers can also identify potential upcoding, undercoding, or misuse of modifiers. This aids them in targeting specific areas for auditing and staff training and also stays compliant with evolving coding guidelines and payer policies.
Payer analysis
Healthcare providers can organize denials by payer, identifying those with disproportionately high denial rates or frequent requests for extensive documentation. Claim analytics reveals underperforming payers in the contract mix and supports contract performance monitoring. Providers can leverage data to negotiate changes in terms or processes, enabling stronger contracts that hold payers accountable, reduce administrative burdens, and foster a more balanced reimbursement environment.
Denial management and prevention
Claims denials occur for various reasons, from missing referrals to incomplete prior authorizations. Kaiser data shows that about 10% of claim denials in marketplace plans result from missing referrals or authorizations, while 16% arise due to service exclusions in the patient’s plan.
Claims analytics can provide a clear understanding of which factors drive these denials, allowing RCM teams to make proactive adjustments. Providers can invest in areas that require external support, strengthening the practice’s ability to reduce denials and protect revenue.
Revenue cycle optimization
Claims analytics set up automated checks, refine billing protocols, and streamline workflows to minimize delays. Healthcare providers can improve documentation, implement staff training, enhance coding accuracy, or reduce denied claims. This improves clean claims submission, increases claim acceptance rates, and accelerates reimbursement cycles.
Fraud detection and prevention
Claims analytics is highly effective at spotting duplicate claims, upcoding, or unusually high-cost services that do not match patient diagnoses. These red flags enable providers to detect fraud early and take corrective action. Providers can eliminate unnecessary services or excessive charges, adopting a proactive approach that protects them from legal risks and costly penalties.
How to implement a claims analytics strategy
The potential of data mining and claims analytics can transform healthcare provider's approach to claims management. However, simply conducting claims analysis won't achieve the results. Here's a step-by-step guide to building an effective claims analytics strategy for sustained improvement.
Assess current processes
Start by evaluating existing claims management processes to identify areas with the greatest opportunity for improvement. Conduct a detailed workflow analysis or create a process flowchart to map out each step of the claims journey. This visual representation aids healthcare providers in uncovering inefficiencies or bottlenecks. By understanding the starting point, practices can approach claims analysis with clarity and better target process changes.
Set KPIs
Establish measurable KPIs based on industry benchmarks or set internal goals that reflect priorities. Decide on specific metrics, such as reducing the claims cycle time, improving your settlement ratio, or boosting customer satisfaction. By tracking KPIs over time, healthcare providers will be able to gauge the impact of their strategy and make data-driven adjustments as needed.
Pool existing data and ensure compatibility
Gather all relevant data sources to support analytics initiatives and address any issues with compatibility or data accessibility. Data silos can significantly slow down analytics capabilities. Aim to standardize data collection methods and secure datasets to maintain data quality and compliance.
Although some advanced software solutions can process unstructured data — such as written records or images that haven't been digitized — a standardized approach to data management will streamline the analytics process and lead to more reliable insights.
Implement tailored software solutions
Select a claims analytics software solution that fits specific needs. For instance, if a healthcare provider's focus is on workers' compensation claims, look for software with features like incident tracking; consider PCH Health for seamless processing of various claims types, from workers' compensation to product liability and warehouse incidents. With its user-friendly interface, PCH Health can simplify claims management processes without requiring any technical coding knowledge.
Review, refine, and continue optimizing
Regularly review claims analysis results and use insights from analytics to make ongoing adjustments. This approach allows healthcare providers to refine their claims process for maximum efficiency continually. Whether they're streamlining workflows, improving claim accuracy, or enhancing customer experience, a data-driven strategy will help healthcare providers make informed decisions in real time.
Challenges in claims analytics and how to overcome them
Healthcare providers often face data quality, system integration, and data security concerns. Here’s a look at these challenges and strategies to overcome them.
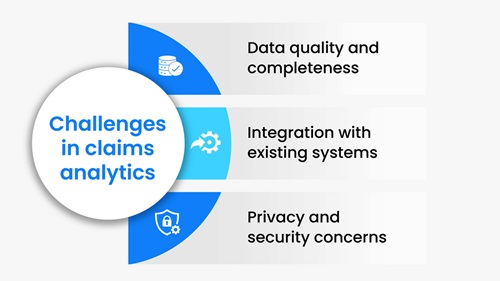
Data quality and completeness
High-quality, complete data is critical for accurate insights. Poor data quality, such as incomplete or incorrect claims data, leads to misleading analytics results, misinformed decisions, and flawed predictive models. Inconsistent data formats and coding errors also reduce the reliability of claims analytics.
Healthcare providers can implement data validation processes to identify and correct errors before analysis. Regular audits of claims data, consistent use of standard coding systems (e.g., ICD-10, CPT codes), and staff training on accurate data entry are essential practices. In addition, AI-enabled data cleansing tools can automatically detect and correct inaccuracies, improving data reliability.
Integration with existing systems
Healthcare providers often rely on a variety of systems, including electronic health records (EHR), practice management software, and billing platforms. Integrating claims analytics solutions with these systems can be challenging due to data silos, differing data structures, and lack of interoperability standards. Fragmented data sources slow down analytics processes and hinder access to a unified view of claims data.
To facilitate integration, organizations should prioritize solutions that adhere to interoperability standards like HL7 and FHIR. Working with vendors who offer flexible APIs (Application Programming Interfaces) and compatibility with common healthcare platforms can simplify integration. Conducting a phased implementation and involving IT and revenue cycle teams in planning helps to identify and mitigate potential issues early, ensuring smoother integration.
Privacy and security concerns
Claims data contains sensitive patient information, and mishandling it can lead to severe privacy violations. Strict adherence to regulations like HIPAA is essential to avoid unauthorized access, data breaches, and potential legal consequences. Privacy and security concerns also heighten as more data is aggregated across systems for analytics.
To ensure data security, healthcare providers should implement encryption protocols for data both in transit and at rest. Access controls that limit data access to authorized personnel, regular security audits, and robust data governance policies are crucial for maintaining compliance. Adopting cloud-based analytics platforms with robust security certifications (such as HITRUST or SOC 2) further strengthens data protection.
Future of claims analytics
Advancements heavily influence the future of claims analytics in AI and machine learning. These technologies help healthcare providers detect denial patterns, identify fraud, and anticipate high-risk cases that require early intervention. By automating routine tasks, like coding and documentation checks, AI reduces manual errors and accelerates the claims cycle, freeing staff to focus on more complex cases and strategic tasks.
Real-time analytics and fully automated claims processing are on the horizon. These will allow providers to immediately address issues as claims are generated, significantly reducing denials and improving cash flow. The increased adoption of predictive analytics, combined with automation, will empower healthcare organizations to proactively manage costs, allocate resources more efficiently, and ultimately deliver a higher quality of patient care.
Are you looking for effective claims analytics solutions? Connect with PCH Health. We harness the power of claims analytics to help healthcare providers achieve growth. Our solutions, powered by advanced AI and machine learning technologies, are designed to optimize your revenue cycle, reduce denials, and improve overall operational efficiency. Click here to explore how claims analytics can transform your organization and elevate both financial and clinical outcomes.